Artificial intelligence and data
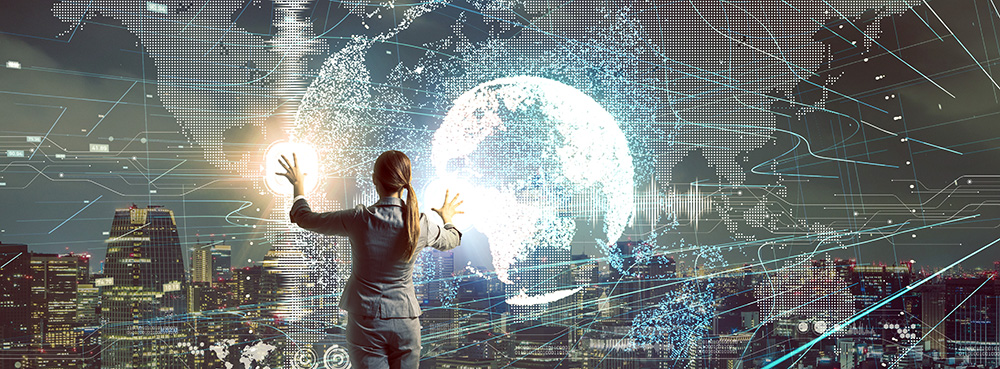
Preamble
The AI and data revolution is on the move. It is no longer at our doorstep, it has crossed the threshold and started to take hold in our living rooms. So the issue of the businesses that are accompanying it, and that will accompany tomorrow’s world, is essential: what are the jobs offered by AI and data? How to prepare for them?
Not that old story again! Granted. Yet now, it only takes a few clicks to get a wealth of answers. You will hear abundantly about data scientists, data engineers, data managers, data scientists, data analysts, machine learning engineers, or even data Architects.
More commonly discussed in English, artificial intelligence (AI) and data give the feeling that they are the sole preserve of specialists.
But then, what about questions that seem to be coming from all sides: how to develop a business approach to AI if it is only driven by a technical approach? How to think about AI’s organizational consequences in companies if it is a matter for sole specialists? How to convince those who are “far from AI” that it generates benefits, not only anxieties? How to reconcile business units and professions with research and development (R&D) or with information systems (IS) managers? And more generally: how can we democratize its use and learn new skills if it is agreed in advance that we will never ever have a clue about it?
With the list of previous professions as a starting point, the answers seem uncertain. Three specialists in AI and data, David Cressey, Frédéric Oru and Boris Yepmo, have agreed to answer our questions related to AI and data business research.
David Cressey
Head of BeautyTech Accelerator - EMEA - Data & AI chez L'Oréal
Membre du Board d’aivancity
Frédéric Oru
Professeur Expert, Mathematics for AI & Data Science
Boris Yepmo
Professeur Expert, Data science et Python
“Technical” point of view: Technique is everything!
This statement sounds like an obvious one. In France, apart from engineering schools, specialized schools and high-level master’s degrees, there’s no way to get ahead. And it’s quite true: understanding machine learning, gradient regression, neural networks convolution or the curse of dimensionality at the very least, presupposes that one understands the words used, that one knows what “learning”, “gradient”, “convolution”, “neurons”, “dimensionality” mean...
No salvation in the land of AI and data without a solid mathematical and computer science foundation. Don’t AI experts all come from the same schools? From the same demanding training courses? Through the same study paths?
On dispose même d’une carte pour se diriger aux pays des métiers de l’IA et de la Data1 :
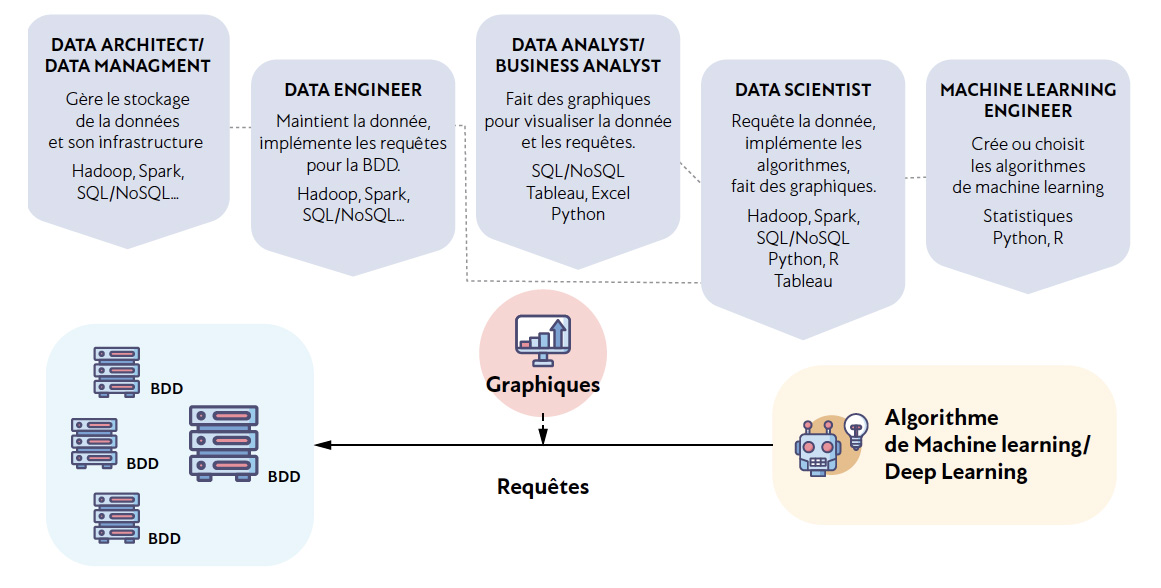
Our 3 specialists were quick to react
Boris Yepmo, is the first to react, weighing his words:
“This breakdown is very specific to the Tech. For a company that is more business-oriented, the functions will not be the same! Profiles have changed: you need very advanced knowledge in both fields, Tech and business.
David Cressey, is more didactic:
“I don’t think this map is wrong. Nevertheless, as the field is still quite immature in non-tech companies, I’d rather talk about an ecosystem of skills that evolves within ta threefold field: data, business, IT. The roles that are mentioned in this diagram are a subset of what is found in organizations today, but they are likely to evolve rapidly. However, to conclude, I am not sure I understand the purpose of this map and I recommend not to use it as it stands!”
Frédéric Orru agrees :
“that map only addresses the ‘technical’ profiles of AI and data. It would be necessary to fill in the picture with the IT operating profiles of the ISD (management of servers, connections, etc.), access rights to APIs, IS integration ...) and the business profiles that work with the data analysts and data scientists (but these are “frontier” jobs that are not yet fully mapped) and jobs in the making, such as AI auditor, ethics manager, etc... “
So another map of professions, another landscape do exist indeed! So the technical specialists in AI and data were missing something, but what was it? We had to turn the question around and no longer ask what we needed from a “technical” point of view for an AI project, but what we needed from a “business” point of view for the same project!
Business point of view : Technique is not everything!
“But then, dare I ask, from a business point of view, where are AI and Data specialists found wanting?”
Boris, goes straight to the point:
“The ability to imagine what the projects that will be implemented will be used for. What is created must be suited to market needs. It is necessary to have a vision of the market before starting to produce, so as not to repeat unsuitable inventions such as the Smartphone-connected mug.
Similarly, for the autonomous vehicle, a major innovation in the transportation sector: its development is linked to local or governmental institutions that will have to (re)think the ecosystem these vehicles will run in”.
David, always the didactic one:
“It’s the link between these three skills (business, data, IT) that enables us to create value (ROI, operational efficiency...) within companies. Even if there is no pre-established model, it is interesting to observe that one organizational scheme is becoming more and more widespread: a decentralized data science sector. Indeed, it is believed that a data scientist must be as close as possible to the business in order to become an SME (Subject Matter Expert). Without this skill, it is difficult for him/her to provide the level of value expected by the profession.
We’ve got to keep in mind that data science and AI are only of interest to companies if they enable them to leverage business use cases with the resultant ROI, operational efficiency, customer experience or the development of new business models. An intimate knowledge of the sector, the issues and strategic priorities of the industry and the company are essential assets for data practitioners.
Frédéric completes:
“What I notice is that AI and data specialists often lack knowledge about the businesses they serve, and are not always very good at motivating operational staff to work with them.
This is a fundamental problem: to make good AI, you don’t just need data, you need quality data, that is representative of reality (as a statistical sample), and whose origin and significance are well-understood ...
However, those who produce the data know where it comes from and what it means: they are the people in the trade, the operational people. Data engineers can’t put themselves entirely in their shoes, but they must have a minimum understanding of the business, and an ability to talk to people in the business.”
And he goes on saying:
“But I also have a second point, which concerns the awareness of the impact of his action. Engineers are generally passionate about their technologies but are not much aware of the social or environmental consequences of what they produce”.
Yet AI can have significant effects on jobs, on climate (owing to its energy consumption), on users’ behavior (filter bubble in social networks for example) ... It is necessary to give a more important part to the “humanities” in AI and data specialists training curricula, and I will personally push for philosophy. When you code an AI, you don’t really code a program anymore, you code an intention, an objective. For example: the objective “to hold the reader’s attention” can lead AI to propose conspiracy contents – false obviously, but terribly attractive. Is this really what we want?”
The Villani report is worth reading again: it was one of the first to state, “[AI ethics] Teaching is almost absent from engineering school curricula or university computer science courses, even though the volume and complexity of the ethical issues that these future graduates will be confronted with is constantly growing”.
While the business point of view is essential, “how can you train for these new professions when you don’t have a basis in mathematics or computer science?”
Boris continues:
“It’s entirely possible if the trainer adapts. In order for a novice to acquire the (indispensable) basics of mathematics, he must be immersed in concrete daily life cases”.
Frédéric makes a commitment:
“Without a computer or mathematical base, we can still be trained to understand how AI works. It’s very important to understand that it’s not magic and that it can’t do everything.
If you have a basic knowledge of computer science (and more specifically algorithmic programming), it is quite easy to learn how to train AIs that have well-known models, typically neural networks on images. But for a professional use, the person must understand what they are doing. This requires some basic statistics, but it does not require a PhD in mathematics.
Finally, if you have a basic knowledge of mathematics but little computer science, you understand everything but you don’t know how to do anything. There are a lot of tools to master (Linux, Python, data science libraries, a bit of cloud computing, big data tools, versioning tools) ... taken in isolation it’s not complicated but it’s still a lot of material to remember”.
David, is anxious to come back to the company, and points out :
“It is very useful to quickly bring on board, from the very beginning of the project, people capable of building robust business cases in order to estimate the potential gains related to an AI project. These profiles must have an intimate knowledge of the company’s business, but also of the notions of corporate finance. A layer of knowledge in tech / AI will be necessary for them but it can be acquired quickly”.
New jobs? What new jobs?
To conclude, before proposing a new map, what about the new professions related to matters of trust and ethics? What employability? AI and Law, AI and Culture, AI and Health, AI and journalism, AI and Sport, AI and customer relations?
Boris concludes :
“All fields are and will be impacted: AI already prescribes our cultural tastes, AI targets journalistic information ... It has clearly penetrated the cultural and information sectors. AI and algorithms are massively used to recommend us content. Also, if the consulting mode is already used in these fields, it should be clearly confirmed in real hiring opportunities within 10 years...”
David is kind enough to continue :
“Ethics is at the heart of the concerns of companies that plan to grow thanks to AI. This is especially true when developing AI tools that interact or have a direct impact on a company’s customers (credit granting, image recognition, scoring marketing...).
As far as law, culture and journalism are concerned, I believe that no field can claim to be insensitive to AI developments. It is up to specialists in these fields to embrace new technologies to shape them in a way that maximizes the benefit to society as a whole rather than letting a few technological giants dictate the future of their specialties”.
Frédéric concludes :
“I was talking about it a little earlier: these jobs are in the making. My feeling is that there will be more and more market demand, if only for regulatory reasons. It would still be difficult, in my opinion, to define a training path today that produces specialized “AI and Law”, “AI and Culture”, “AI and Journalism”, “AI and Sport” profiles, etc. On the other hand, it is clearly possible to create dual curricula in connection with law schools, art schools, schools of journalism, to bring them a sound AI culture... and why not invent the foundations of their future jobs together with them?”
Epilogue
Taking up their words, we can imagine the following table:
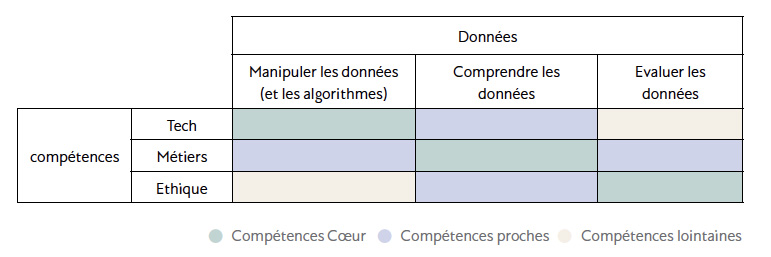
Handling data – in the mathematical sense – and algorithms is the core competence of technical professions.
Understanding data – in the sense of “knowing where it comes from and what it means”, to paraphrase Frédéric – is the core skill of technical professions.
Finally, evaluating data – in the sense of assessing the stakes and impacts from a societal and regulatory perspective – is the core business of the DPO or of the legal operations officer, or even of the “legal operations officer”, or then again of the SRC manager.
With this short diagram, the AI professions could be distributed according to a triptych of skills related to the type of data use that was sought. While a “data scientist” ’s core competence will never be data assessment – from the point of view of its societal and regulatory issues – he or she cannot ignore this area.
Finally, let’s propose another map, that of approaches for the success of an AI project:
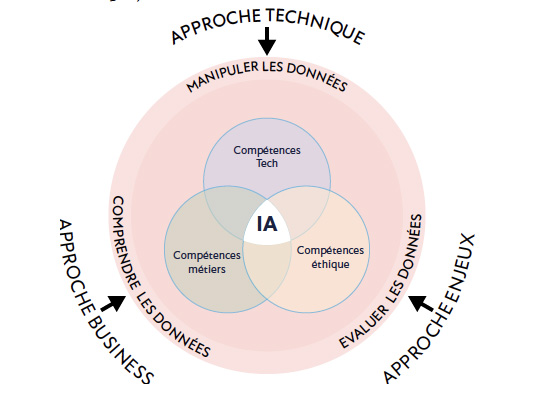
What would our three specialists think? Makes you wonder....
Christophe de Beauvais
Strategic Advisor to aivancity